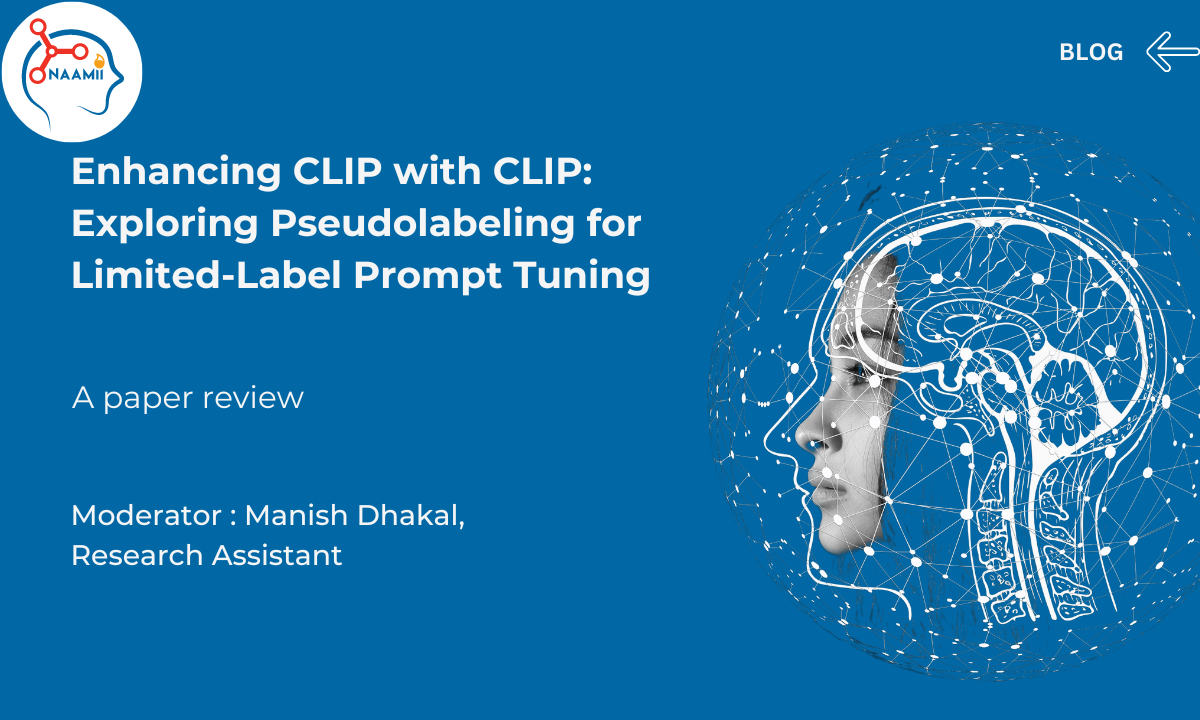
A paper review on “Enhancing CLIP with CLIP: Exploring Pseudolabeling for Limited-Label Prompt Tuning”
- Post by: Naamii
- January 23, 2024
- No Comment
Co-authors and Affiliations: The paper is co-authored by Cristina Menghini, Andrew Delworth, and Stephen H. Bach, all affiliated with CS at Brown University.
Link to the Original Paper: You can read the full paper at https://arxiv.org/abs/2306.01669
Summary of the Research: With iterative approaches for generating the pseudo-labels dynamically from the zero-shot classification capabilities of the CLIP, we can effectively fine-tune prompts for down-stream tasks irrespective of their modality (unimodal or multimodal) and learning paradigms – semi-supervised learning (SSL), transductive zero-shot learning (TZSL), and unsupervised learning (UL) paradigms.
Problem Statement: The paper addresses the domain of limited or zero labeled data, and larger pool of unlabeled data. However, vision-language models (VLMs) such as CLIP already exist in the literature which can be leveraged to generate pseudolabels of the unlabeled data. This paper bridges the gap between limited data training and zero-shot capabilities of the VLMs.
Methodology: First, the paper proposes a method of generating the pseudolabels from VLMs and using to finetune the prompts of CLIP model for the specific classification tasks. The top-K pseudolabels for each input image are used in this generation step to mitigate the class imbalance problem. Secondly, a unified objective function is defined that can be used for all the three learning paradigms mentioned above. Lastly, the paper proposes two main strategies of getting pseudolabels: (1) use static pseudolabels for a single training experiment, and (2) dynamically update pseudolabels for each training iteration from the checkpoint of previous iteration of the finetuned CLIP model.
Conclusions and Differentiating Factors: The paper exhibited that using the dynamically generated pseudolabels of all the unlabeled data gives significant improvement in all the learning paradigms and across all modalities mentioned above.
Moderator’s Note: Basing on the methods used for generating pseudolabel, I believe that we can leverage BiomedCLIP model to generate the pseudolabels for medical images. This paper gave us a deeper understanding of the prompting methodologies in both unimodal and multimodal inputs in VLMs when the encoders’ weights are kept unchanged.
About the moderator: The moderator for this paper was Manish Dhakal, a research assistant at NAAMII.
References